How Retailers Use Data to Enhance Inventory Management
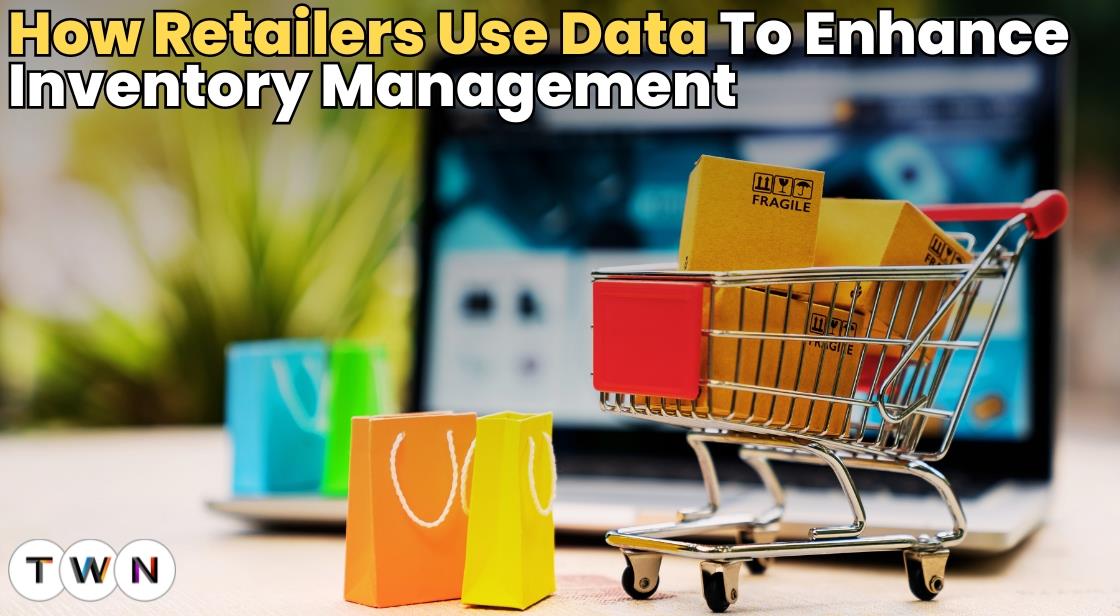
Blog Post
In the ever-evolving world of retail, managing inventory effectively is crucial for maintaining a competitive edge and meeting customer expectations. Traditional inventory management methods, while useful, often fall short of addressing the complexities of modern retail environments.
To stay ahead, retailers need to adopt advanced, data-driven strategies that enhance inventory accuracy, optimize stock levels, and ultimately drive profitability.
One of the most transformative approaches in inventory management is leveraging machine learning (ML) for demand forecasting. ML enables retailers to predict future demand with greater precision, adapt to real-time changes, and shape consumer behavior. Coupled with this, real-time and predictive analytics offer dynamic tools for monitoring inventory and sales trends, reducing overstock and stockouts, and ensuring optimal inventory accuracy.
Furthermore, harnessing historical data through predictive analytics allows retailers to refine their strategies based on past sales patterns, market trends, and customer behavior. Implementing just-in-time (JIT) inventory management and ensuring real-time unification of inventory data across channels are also pivotal in enhancing operational efficiency.
Retailers can also benefit from advanced technologies such as AI-driven shrinkage prevention and geospatial data analysis. By analyzing geographic and demographic information, retailers can tailor their inventory strategies to meet local demands and outperform competitors.
In this blog, we will explore these cutting-edge strategies and technologies, providing insights on how retailers can effectively leverage data to optimize inventory management and achieve sustained success in today's competitive retail landscape.
Data-Driven Strategies for Inventory Optimization in Retail
1. Forecast Demand Using ML
Introduction Forecast Demand Using ML
In today’s competitive retail landscape, merely analyzing data is insufficient. Retailers must go beyond traditional methods to effectively manage inventory and enhance consumer engagement. Forecasting demand with machine learning (ML) emerges as a crucial strategy in achieving these goals. This approach not only predicts future demand but also helps in shaping it, giving retailers a significant edge over competitors.
The Importance of Demand Forecasting
Accurate demand forecasting allows retailers to align their inventory with consumer needs, minimizing overstock and stockouts. By leveraging ML, retailers can analyze vast datasets and identify patterns that traditional methods might miss. This enables them to make more informed decisions about inventory levels, promotions, and supply chain logistics.
How Machine Learning Enhances Forecasting
Machine learning models improve demand forecasting by analyzing historical sales data, market trends, and external factors like seasonal variations and economic conditions. These models use algorithms to predict future demand with high precision, adapting to changes in real-time. This dynamic approach allows retailers to respond swiftly to shifting consumer preferences and market conditions.
Shaping Demand Through Forecasting
Forecasting with ML not only predicts demand but also influences it. By understanding consumer behavior and market trends, retailers can tailor their marketing strategies, adjust pricing, and optimize inventory placement to drive desired demand. This proactive approach helps in maximizing sales and improving customer satisfaction.
Also Read: Consumer Psychology: How to Understand and Predict Customer Desires
2. Dynamically Monitor Inventory And Sales Trends
Real-Time Data Analytics
Retailers should embrace real-time data analytics to keep a continuous watch on inventory levels and sales trends. By using advanced analytics tools, they can access up-to-the-minute information about stock availability and sales performance, allowing them to make informed decisions quickly. This real-time monitoring helps in identifying trends and patterns as they emerge, enabling proactive management of inventory.
Predictive Analytics for Optimization
Leveraging predictive analytics is crucial for optimizing stock levels. Predictive models analyze historical data and current trends to forecast future demand, helping retailers adjust their inventory accordingly. This foresight helps in balancing inventory levels—minimizing the risk of overstocking items that may not sell and preventing stockouts of popular products. By accurately predicting demand, retailers can ensure they have the right amount of stock to meet customer needs without excess.
Reducing Overstock and Stockouts
Effective use of real-time and predictive analytics contributes to reducing overstock and stockouts. Overstocking can lead to unnecessary holding costs and markdowns, while stockouts can result in lost sales and dissatisfied customers. By maintaining optimal inventory levels, retailers can enhance their operational efficiency, reduce waste, and improve customer satisfaction.
Improving Inventory Accuracy
Dynamic monitoring of inventory and sales trends enhances overall inventory accuracy. Accurate inventory data ensures that retailers can track stock levels precisely, adjust orders as needed, and respond swiftly to changes in market demand. This accuracy supports better decision-making and strengthens the retailer’s ability to meet customer expectations effectively.
3. Power Predictive Analytics With Historic Data
Retailers today are harnessing the power of predictive analytics to refine their inventory management strategies. By utilizing historical data, they can gain valuable insights into customer preferences and market trends, leading to more informed decision-making.
Leveraging Historical Sales Data
Predictive analytics starts with a deep dive into historical sales data. By analyzing past sales patterns, retailers can forecast future demand with greater accuracy. This involves examining factors such as seasonal trends, promotional impacts, and purchasing behaviors. Understanding these patterns helps retailers anticipate what products will be in demand and when.
Understanding Market Trends
In addition to historical data, market trends play a crucial role in predictive analytics. Retailers analyze broader market conditions, including economic indicators and competitor activities, to refine their inventory forecasts. This helps in aligning inventory levels with anticipated market shifts, ensuring that stock levels are adjusted proactively.
Customer Behavior Analysis
Customer behavior analysis provides insights into individual purchasing patterns. By examining data such as buying frequency, preferences, and feedback, retailers can tailor their inventory to meet specific customer needs. This personalized approach helps in predicting which products will be popular and at what times, further enhancing inventory efficiency.
Implementing Just-in-Time Inventory
With insights from predictive analytics, retailers can implement just-in-time (JIT) inventory management. JIT involves stocking items only when they are expected to be in demand, thus minimizing excess inventory and reducing waste. This approach not only improves inventory turnover but also reduces holding costs and optimizes storage space.
4. Ensure Real-Time Unification Of Inventory Data
The real-time unification of inventory data has become essential in today’s retail landscape. This approach ensures that all inventory information is consolidated into a single, accurate source of truth, providing retailers with a comprehensive view of their stock across various channels.
Rise of Omnichannel Shopping
The need for real-time data unification first gained prominence with the rise of omnichannel shopping. As consumers increasingly expect a seamless shopping experience across physical stores, online platforms, and mobile apps, retailers must integrate their inventory data to meet these expectations.
Increased Complexity Due to Omnipresent Shopper Engagement
The challenge has intensified with the widespread engagement of shoppers through various platforms. Retailers now need to monitor and manage sales not only from their own stores and digital properties but also from third-party services, including social commerce and numerous delivery platforms. This complexity requires an up-to-date, unified inventory system to accurately track and manage stock levels.
Benefits of Unified Inventory Data
Real-time unification allows retailers to maintain accurate inventory records, minimize stockouts and overstocks, and improve customer satisfaction. By having a single source of truth, retailers can make informed decisions, optimize their inventory levels, and streamline their operations.
5. Identify And Promote Products With Flagging Sales
Retailers face the challenge of fluctuating consumer preferences, which can lead to products with declining sales. To address this issue, it’s crucial to analyze inventory data to identify which items are not selling as well as expected. By monitoring sales trends and inventory turnover rates, retailers can pinpoint products that are flagging in terms of sales.
Analyzing Inventory Data
Effective inventory management starts with thorough data analysis. Retailers should regularly review sales data to track the performance of each product. Key metrics include sales velocity (the rate at which products are sold), stock levels, and time on shelves. When a product’s sales start to slow down, it becomes critical to act swiftly to prevent accumulating unsellable stock.
Implementing Creative Promotions
Once slow-selling products are identified, retailers need to develop creative promotions to boost their sales. Promotional strategies might include discounts, bundling with other popular items, or targeted marketing campaigns. These tactics can help attract consumers and encourage quicker sales of the flagged products.
Taking Corrective Actions
In addition to promotions, retailers should consider other corrective actions if sales continue to lag. This could involve adjusting inventory orders, removing the product from shelves, or re-evaluating the product’s market fit. By staying proactive and responsive to sales trends, retailers can manage inventory more effectively and reduce the risk of unsold stock.
6. Set Up A Control Tower For E2E Visibility
Importance of End-to-End Visibility
Establishing a control tower is crucial for achieving comprehensive visibility across the entire supply chain. It allows businesses to monitor and manage every aspect of their operations, from inventory management to supply and demand tracking. This holistic view is essential for optimizing processes and ensuring operational efficiency.
Tracking Inventory and Supply Chain
A control tower enables real-time tracking of inventory levels and supply chain activities. By integrating data from various sources—such as order management systems, inventory databases, and warehouse management systems—businesses can gain a clear understanding of their inventory status, order fulfillment progress, and supply chain disruptions. This visibility helps in identifying potential bottlenecks and making informed decisions to streamline operations.
Enhancing Decision-Making with Analytics
Incorporating analytics and artificial intelligence into the control tower further enhances its capabilities. Advanced analytics provide actionable insights by analyzing historical data and current trends. AI-driven tools can predict future demand, optimize inventory levels, and automate routine tasks. This integration makes data searches easier and supports more accurate and timely decision-making.
Benefits of a Control Tower
Implementing a control tower with these features not only improves operational efficiency but also enables better coordination between different departments. The result is a more agile and responsive supply chain, capable of adapting to changing market conditions and customer demands.
7. Optimize Warehouse Layouts
Introduction to Data-Driven Warehouse Optimization
To improve warehouse efficiency, adopting data analysis to optimize layouts is crucial. Analyzing patterns in item picking can provide valuable insights that enhance the organization of storage spaces. By leveraging data, warehouses can streamline operations, reduce errors, and increase overall productivity.
Analyzing Item Picking Patterns
Data analysis involves examining which items are frequently picked together. By identifying these patterns, warehouses can place these items in proximity to each other. This strategic arrangement minimizes the distance pickers need to travel, thereby speeding up the order fulfillment process.
Strategic Placement of High-Value and Fast-Moving Stock
High-value and fast-moving items should be positioned near packing stations. This placement facilitates quick access, reducing the time spent retrieving these critical items. By optimizing the location of such stock, warehouses can enhance efficiency and responsiveness to high-demand products.
Benefits Beyond Time and Space Savings
While saving time and space is a key benefit, data-driven layout optimization also contributes to a more organized and efficient workspace. It results in happier workers due to reduced physical strain and fewer errors in order picking. Overall, this approach fosters a more productive work environment and improves operational accuracy.
8. Segment Customers To Tailor Inventory
Segmenting customers based on their buying behaviors and purchasing patterns is crucial for effective inventory management. By categorizing customers into distinct groups, such as frequent buyers, seasonal shoppers, or high-value customers, retailers can better understand demand trends for each segment. This segmentation helps in predicting which products are likely to be in demand at different times, allowing for more accurate inventory forecasting.
Tailoring Inventory to Meet Specific Needs
Personalizing inventory based on customer segments ensures that stock levels align with the preferences and needs of each group. For example, retailers can stock up on high-demand items for frequent buyers while offering specialized products for niche segments. This approach reduces the likelihood of overstocking items that may not sell well and minimizes the risk of stockouts for popular products.
Enhancing Replenishment Strategies
With a clear understanding of customer segments and their purchasing patterns, retailers can implement more precise and timely replenishment strategies. Data-driven insights allow for adjustments in inventory levels, ensuring that popular items are restocked quickly while slower-moving products are reduced. This leads to more efficient inventory turnover and better alignment with actual customer demand.
Reducing Overstocking and Stockouts
Effective customer segmentation and inventory personalization directly contribute to reducing overstocking and stockouts. By accurately forecasting demand and tailoring stock levels, retailers can maintain optimal inventory levels, improving customer satisfaction and operational efficiency. This approach not only enhances the shopping experience but also supports better financial performance.
9. Analyze Inventory, Surveillance And POS Data To Prevent Shrinkage
Retail shrinkage, the loss of inventory due to theft or fraud, poses a significant challenge for retailers. To combat this issue effectively, leveraging advanced technologies such as AI and machine learning is becoming increasingly crucial.
AI-Driven Shrinkage Prevention
Implementing AI-driven solutions allows retailers to analyze vast amounts of data from various sources to prevent shrinkage. Machine learning algorithms can process and interpret point-of-sale (POS) data, surveillance footage, and inventory records to detect patterns and anomalies that may indicate theft or fraud.
Analyzing Point-of-Sale Data
POS data provides valuable insights into sales transactions and inventory levels. By analyzing this data, AI systems can identify irregularities such as unusual purchase patterns or discrepancies between sales and inventory records, which may signal potential fraud or theft.
Surveillance Footage Analysis
AI can enhance surveillance by analyzing video footage from store cameras. It can identify suspicious behaviors, such as individuals loitering or acting in ways that deviate from typical customer behavior. Real-time alerts can be generated to address suspicious activities promptly.
Inventory Records Examination
Regular analysis of inventory records using machine learning helps in tracking discrepancies and detecting potential theft. AI systems can pinpoint irregular patterns in stock levels and alert store managers about discrepancies that need investigation.
Optimizing Store Layouts
AI can also optimize store layouts and product placements to deter theft. By analyzing data on theft patterns, AI can recommend strategic placement of high-value items and adjust store layouts to reduce opportunities for theft.
10. Use Geospatial Data To Optimize Each Store’s Inventory
Retailers can significantly enhance their inventory management by leveraging geospatial data analysis. Geospatial data involves information related to geographic locations and is instrumental in optimizing inventory levels for each store based on its specific location. This approach helps in tailoring inventory strategies to meet local demands more effectively.
Analyzing Demographic Data
Geospatial data analysis begins with examining demographic information relevant to each store's location. Understanding the age, income level, and lifestyle of the local population allows retailers to stock products that align with the preferences and needs of the community. For example, a store in a high-income area might stock luxury items, while a store in a family-oriented neighborhood could focus on household essentials.
Evaluating Local Economic Conditions
Local economic conditions, such as employment rates and economic growth, also play a crucial role. By analyzing these factors, retailers can adjust their inventory to reflect the purchasing power of the local population. Stores in economically thriving areas may benefit from a broader range of premium products, while those in less affluent regions may focus on more budget-friendly options.
Assessing the Competitive Landscape
Understanding the competitive landscape is another key aspect. Geospatial data can reveal the presence of competitors and their offerings, allowing retailers to strategically position their inventory to differentiate themselves. For example, if a competing store is known for specific products, a retailer might choose to offer complementary items or unique alternatives to capture market share.
Tailoring Inventory Strategies
By integrating these geospatial insights, retailers can optimize inventory levels for each store, ensuring they stock the most relevant products for their specific geographic area. This not only reduces excess inventory but also improves sales and customer satisfaction by aligning product offerings with local demand.
In conclusion
Data-driven strategies are essential for modern inventory management in retail. By forecasting demand with machine learning, monitoring trends in real-time, leveraging historical data, unifying inventory systems, and using geospatial insights, retailers can optimize inventory levels and enhance operational efficiency. Implementing these strategies helps reduce overstock and stockouts, improve customer satisfaction, and increase sales. Advanced technologies like AI also play a crucial role in preventing shrinkage and tailoring inventory to specific customer segments. Embracing these data-driven approaches allows retailers to stay competitive and respond dynamically to market demands.
You May Like
EDITOR’S CHOICE