Elon Musk Declares AI Training Has Exhausted Human Knowledge
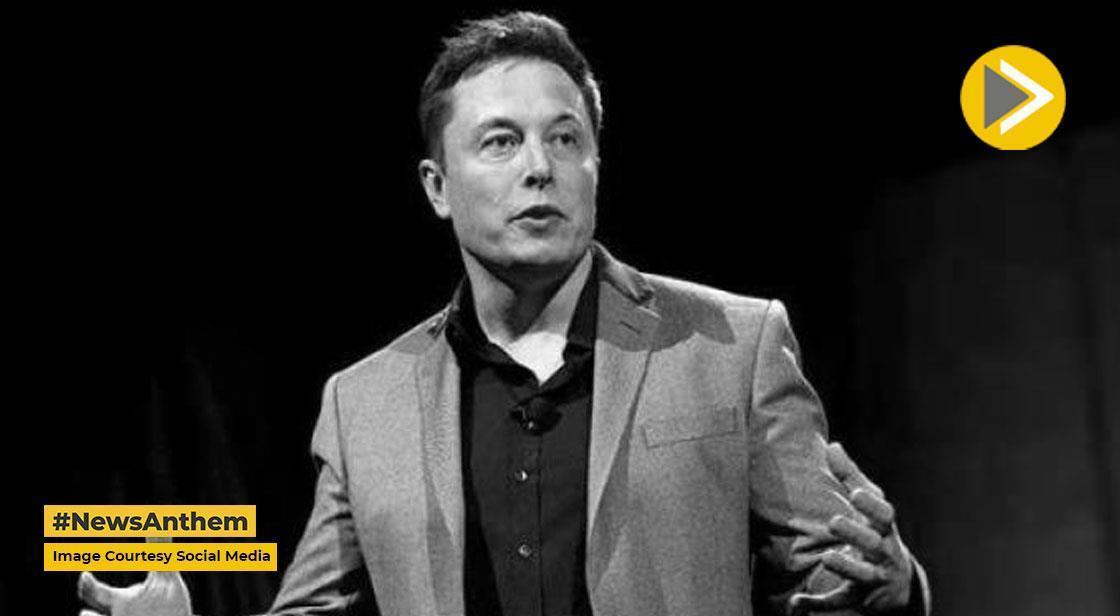
News Synopsis
Elon Musk, the renowned entrepreneur and owner of AI company xAI, has joined other industry experts in affirming a pressing issue in artificial intelligence development—the depletion of real-world data for AI training.
During a live-streamed discussion with Stagwell Chairman Mark Penn, Musk said, "We've now exhausted basically the cumulative sum of human knowledge... in AI training. That happened basically last year." \
This aligns with claims made by former OpenAI Chief Scientist Ilya Sutskever, who declared the industry had reached "peak data" during the NeurIPS machine learning conference in December 2023.
The End of Real-World Data for AI
What Experts Are Saying About "Peak Data"
Ilya Sutskever, a prominent voice in AI, hinted at the limitations of relying solely on real-world data for training advanced AI models. He stated that the scarcity of usable data will necessitate a shift in how AI models are built and refined. Musk, echoing these sentiments, sees synthetic data as the way forward, offering a glimpse into the potential of AI systems training themselves.
Elon Musk's Take on Synthetic Data
A New Paradigm in AI Training
Musk emphasized the importance of synthetic data in overcoming the limitations of real-world data, explaining, "The only way to supplement [real-world data] is with synthetic data, where the AI creates [training data]. With synthetic data ... [AI] will sort of grade itself and go through this process of self-learning." This approach involves AI generating and using its own data to learn, marking a significant paradigm shift in the industry.
Industry Examples of Synthetic Data Usage
Major tech companies like Meta, Microsoft, OpenAI, and Anthropic have already begun incorporating synthetic data in their AI training processes. A Gartner report revealed that 60% of the data used for AI and analytics projects in 2024 was synthetically generated. Notable examples include Microsoft's Phi-4 model and Google's Gemma model, both leveraging a mix of real-world and synthetic data.
Advantages and Risks of Synthetic Data
Cost Efficiency in AI Development
The adoption of synthetic data offers significant cost savings. For instance, Writer, an AI start-up, successfully trained its Palmyra X 004 model primarily on synthetic data at a cost of $700,000. In contrast, a comparable OpenAI model trained with conventional methods would require an estimated $4.6 million.
Risks of Model Collapse
Despite its advantages, synthetic data presents notable challenges. Research suggests that over-reliance on synthetic data may lead to "model collapse," where an AI model's output becomes less innovative and more biased over time. This happens because synthetic data can inadvertently amplify the biases and limitations of the models that generate it, posing risks to the reliability and diversity of AI applications.
The Road Ahead for AI Training
The debate surrounding the exhaustion of real-world data underscores the AI industry's need to evolve. As synthetic data becomes more prominent, balancing cost-efficiency with innovation and fairness will be critical. Musk's perspective and the industry's gradual shift highlight a new chapter in AI's development, where the interplay of human and synthetic intelligence could define the future.
Conclusion
The depletion of real-world data for AI training marks a pivotal moment in the evolution of artificial intelligence. Elon Musk's assertion, supported by other leading AI experts, underscores the urgent need for innovative solutions like synthetic data to sustain AI development. While synthetic data offers cost-effective and scalable alternatives, it comes with its own set of challenges, including potential risks of model collapse and inherent biases.
As the industry pivots towards this new approach, it must strike a delicate balance between leveraging synthetic data's benefits and mitigating its risks. The path forward will require continuous refinement, robust benchmarking, and transparent feedback mechanisms to ensure AI systems remain creative, unbiased, and reliable.
This shift not only highlights the adaptability of the AI community but also signals a transformative phase where machines increasingly train themselves, opening up new possibilities for the future of artificial intelligence.
You May Like